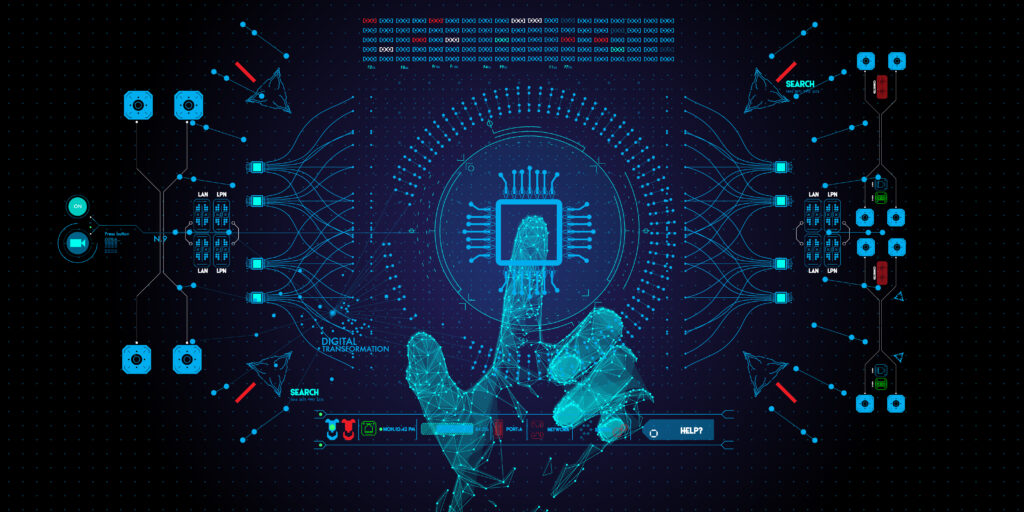
Manufacturers in all industries understand that the key to increased productivity is keeping manufacturing equipment working smoothly and without sudden stoppage. Short stoppages are common, but lengthy downtime for repairs or part replacement is still a danger.
To increase manufacturing productivity, individuals typically focus on increasing the processing capacity of the equipment itself. However, the short stoppages and unexpected shutdowns are more inconvenient. They complicate productivity forecasting and are inconvenient to deal with. Potential advancements in this area would be a significant advantage for smart manufacturing.
Using IoT to prevent the minor delays that accumulate to cause downtime.
Inspection and maintenance are critical for keeping manufacturing lines running safely and efficiently. There is no doubt that this requires time, effort, money, and people, yet certain countries have experienced a maintenance manpower scarcity in recent years due to a dropping birth rate and an older population. Furthermore, because maintenance is indirectly related to product value, many organizations would desire to reduce the number of employees involved.
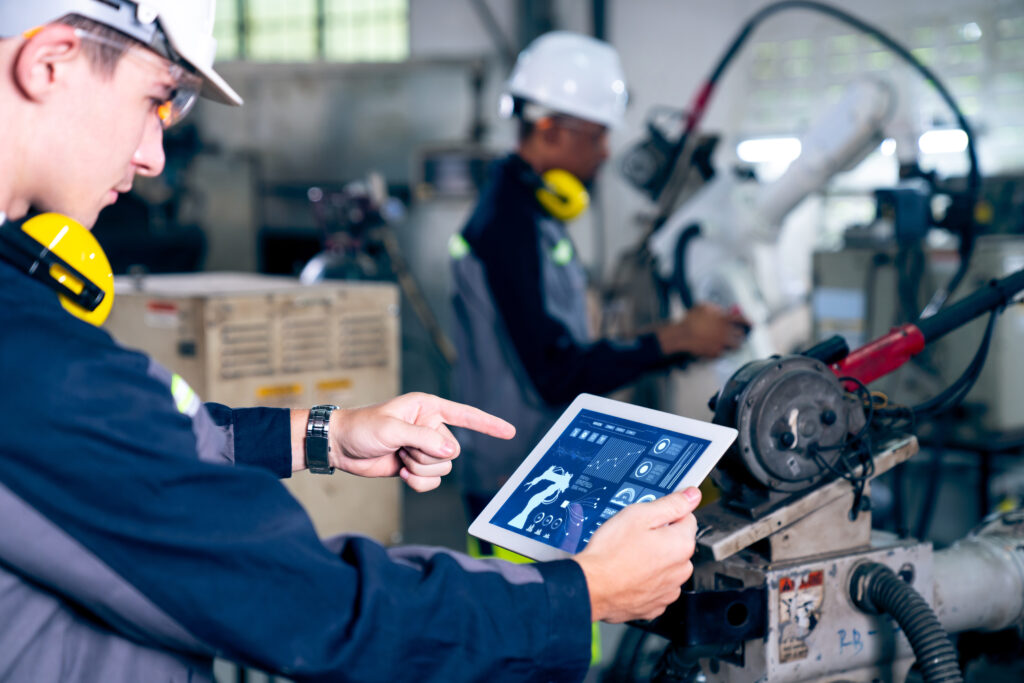
Short stoppages on production lines are caused by a combination of circumstances, such as minor delays, equipment malfunctions, or synchronization issues. Short stoppages are difficult to foresee due to the multitude of factors involved.
Recently, manufacturers have used IoT to collect data on equipment operating conditions and worker movement that can be analyzed in real time. The collection and big-data analysis of the data have resulted in strategies for anticipating short stoppages based on previous incidents. Anticipated short stoppages may be avoided by modifying the working conditions of relevant equipment or involving more staff.
Transforming from Corrective and Traditional Preventive Maintenance Practices
Other issues, such as mechanical parts wearing out or rusting, dirt or foreign matter becoming lodged, or warmer temperatures causing expansion, may require repair. Changes that lead to failure are complicated and gradual, making it difficult to foresee.
Preventive maintenance has generally included attentive frequent inspection and replacement of partially worn consumable parts to avoid failures that necessitate repair. This technique has posed two challenges. First, individual part variation means that failure may occur sooner than anticipated. Second, it appears wasteful to replace perfectly fine components before they reach the end of their useful life.
However, in recent years, IoT and AI have permitted a more proactive approach by detecting early indicators of failure. This is referred to as predictive maintenance. Potential indicators such as appearance, sound, pressure, heat, and vibration are recorded as data from sensors on industrial equipment, and AI-powered analysis algorithms aid in the early detection of failure or malfunction. Consumable parts can be maximized with predictive maintenance. This allows for a methodical approach to maintenance and part orders.
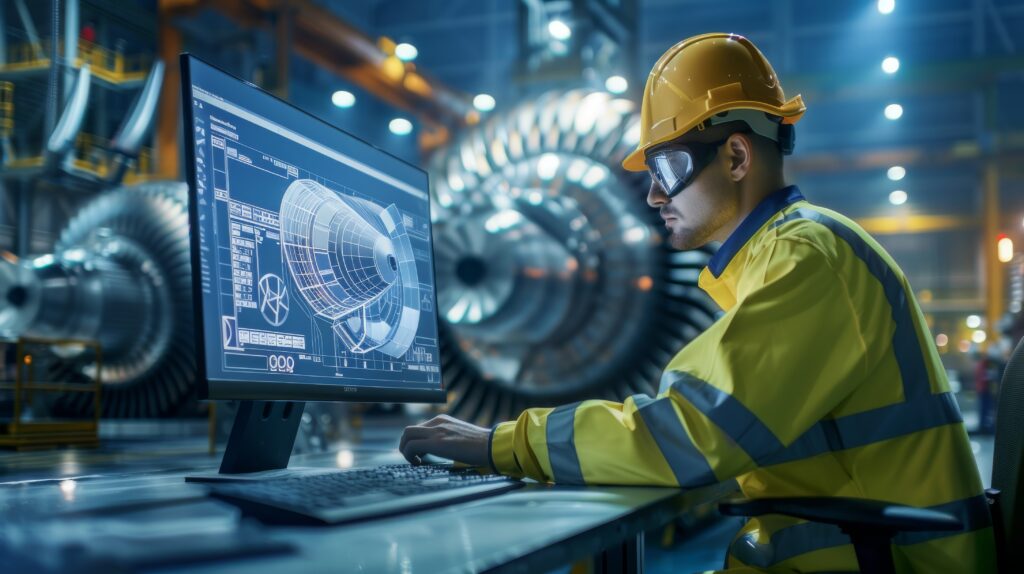
Smart Factories Detect Anomalies with the Precision of Veteran Engineers
At some long-established facilities, experienced engineers can detect equipment irregularities through sound or vibrations felt with their hands. This vigilant awareness has prevented short stoppages or equipment failures that need maintenance.
There are fewer and fewer of these senior engineers, and as the birth rate falls in a graying population, it is difficult to pass on these talents with fewer people to teach. Smart factories provide continuous monitoring of all production equipment without missing anomalies, which is impossible to achieve in traditional factories relying on a small number of skilled workers.
Consistent Yield and Quality Driven by Innovations in Sensors, Communication Modules, and Batteries
More advanced data gathering and analysis technologies with more accurate anomaly detection provide additional benefits than only failure prediction. We can also foresee things like deteriorating yield and quality. However, more effective anomaly detection requires manufacturers to collect enough high-quality data from suitable locations. This also demands for more advanced sensors, communication modules, batteries, and other components of IoT systems that collect the data.
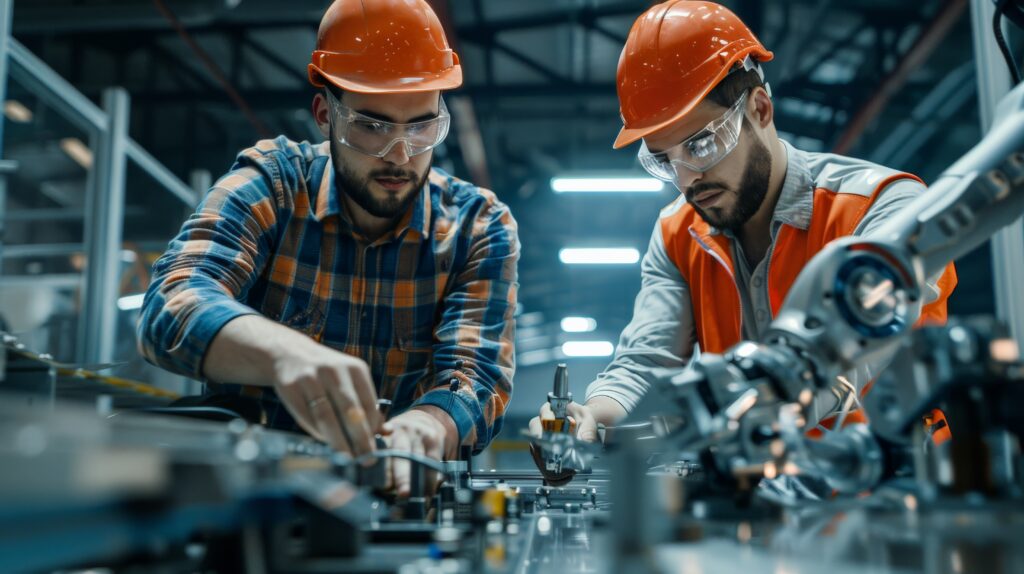
To do this, these components must be made smaller, lighter, and more energy-efficient, so that installation restrictions do not limit data collection locations. To provide reliable functioning even in harsh production circumstances, the components must be highly resistant to environmental factors and noise.
Energy harvesting is a recent discovery that is progressively gaining popularity as a power source for IoT devices. It converts ambient energy sources such as light, temperature differentials, electromagnetic waves, or vibration into electricity. Looking ahead, oxide-based solid-state batteries that improve the environmental resilience of battery-powered IoT devices have the potential to broaden their applications.